Elevators are primarily built from sheet metal parts and components. These sheet metal parts are manufactured by punching and bending raw sheet metal with punching machines. This makes punching machines crucial machines in the manufacturing process of elevators. When unexpected breakdowns happen, turbulence is caused to the manufacturing processes and most likely cause late deliveries for the production orders. The supply unit's goal and ambition are to minimize the punching machines downtimes to guarantee the timely delivery of the elevators to the customers.
The SERENA testbed selected to be implemented to the Finn Power SB8 punching machine. The machine manufactures punched sheet metal parts for different elevator parts. Thus, the testing is in a live production environment. In more detail, the punching machine's monitored components are several bearings of conveyors that transport punched parts forward to the following process and scrap metal from punching to trash bins. Generally, if any component breaks, the machine would be out of use until the failure or breakage is fixed. Therefore, the bearings were selected to be monitoredvia vibration sensors attached to the hull of the bearings. Besides, the punching tool is also monitored. When the punching tool starts to dull after a period of production time, the sheet metal parts' produced quality starts to suffer. Measuring methods used for this are, acoustic emission sensor, microphone, and vibration sensor.
The testbed development included the installation of data collection devices and software, conveyor bearing vibration sensors and punching tool acoustic emission sensor, vibration sensor and microphone. Raw data analysis is performed first in the SERENA cloud, and in the second phase, the analysis is taken to the edge level to be handled by a Raspberry. The results of these fault indicators are delivered to the SERENA cloud and based on this, MIMOSA schema relations are used to create an alert and estimation of the severity of the failure. Next, the scheduling service plans for maintenance activity. The resulted event is enriched with the required maintenance support information and communicated to a maintenance operator at the pre-determined by the scheduler time.
The SERENA solutions were able to improve preventive maintenance scheduling and maintenance resource scheduling including spare parts inventory as well as to decrease down time’s waiting and repair time based on AR solution and spare parts inventory strategy. In particular, SERENA solutions contributed to an increase of MTBF by 100 minutes and a 10% reduction of maintenance costs.
Country: | FI |
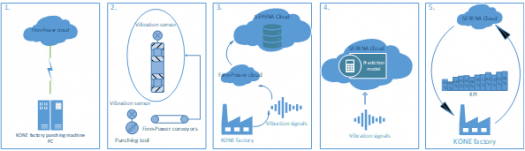
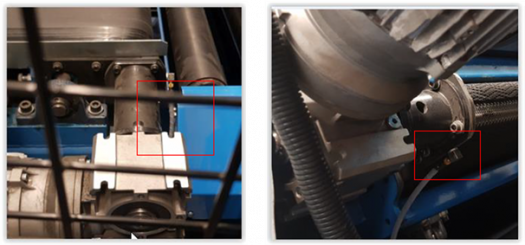
In this case edge analytics performed by a low-cost edge device (Raspberry Pi) proved it is feasible to practice predictive maintenance systems like SERENA. However, performance issues were caused by having a large sampling frequency (16kHz) and background processes running on the device, which affected the measurements. By lowering the sampling frequency, this issue can be reduced. Another way would be to obtain different hardware with buffer memory on the AD-card. In addition, a real-time operating system implementation would also work. It was found that the hardware is inexpensive to invest in, but the solution requires a lot of tailoring, which means that expenses grow through the number of working hours needed to customize the hardware into the final usage location. If there are similar applications thatimplement the same configuration, cost-effectiveness increases. Furthermore, the production operators, maintenance technicians, supervisors, and staff personnel at the factory need to be further trained for the SERENA system and its features and functionalities.