The rise of the system complexity, the rapid changing of consumers demand require the European industry to produce more customized products with a better use of resources.
The main objective of IMPROVE is to create a virtual Factory of the Future, which provides services for user support, especially on optimization and monitoring. By monitoring anomalous behaviour will be detected before it leads to a breakdown. Thereby, anomalous behaviour is detected automatically by comparing sensor observation with an automatically generated model, learned out of observations. Learned models will be complemented with expert knowledge because models cannot learn completely. This will ensure and establish a cheap and accurate model creation instead of manual modelling.
Optimization will be performed and results will be verified through simulations. Therefore, the operator has a broad decision basis as well as a suggestion of a DSS (Decision Support System), which will improve the manufacturing system. Operator interaction will be done by a new developed HMI (Human Machine Interface) providing the huge amount of data in a reliable manner. To reach this aim, every step of the research process is covered by a minimum of two experienced consortium partners, who conclude the results of the project using four demonstrators.
The basis for IMPROVE are industrial use-cases, which are transferable to various industrial sectors. Main challenges are reducing ramp-up phases, optimizing production plants to increase the cost-efficiency, reducing time to production with condition monitoring techniques and optimise supply chains including holistic data. Consequently, the resource consumption, especially the energy consumption in manufacturing activities, can be reduced. The optimized plants and supply chains enhance the productivity of the manufacturing during different phases of production. Furthermore, the industrial competitiveness and sustainability in EU will be strengthened.
Web resources: |
http://improve-vfof.eu/
https://cordis.europa.eu/project/id/678867 |
Start date: | 09-01-2015 |
End date: | 31-08-2018 |
Total budget - Public funding: | 4 148 554,00 Euro - 4 148 554,00 Euro |
Original description
The rise of the system complexity, the rapid changing of consumers demand require the European industry to produce more customized products with a better use of resources.The main objective of IMPROVE is to create a virtual Factory of the Future, which provides services for user support, especially on optimization and monitoring. By monitoring anomalous behaviour will be detected before it leads to a breakdown. Thereby, anomalous behaviour is detected automatically by comparing sensor observation with an automatically generated model, learned out of observations. Learned models will be complemented with expert knowledge because models cannot learn completely. This will ensure and establish a cheap and accurate model creation instead of manual modelling. Optimization will be performed and results will be verified through simulations. Therefore, the operator has a broad decision basis as well as a suggestion of a DSS (Decision Support System), which will improve the manufacturing system. Operator interaction will be done by a new developed HMI (Human Machine Interface) providing the huge amount of data in a reliable manner.
To reach this aim, every step of the research process is covered by a minimum of two experienced consortium partners, who conclude the results of the project using four demonstrators.
The basis for IMPROVE are industrial use-cases, which are transferable to various industrial sectors. Main challenges are reducing ramp-up phases, optimizing production plants to increase the cost-efficiency, reducing time to production with condition monitoring techniques and optimise supply chains including holistic data. Consequently, the resource consumption, especially the energy consumption in manufacturing activities, can be reduced. The optimized plants and supply chains enhance the productivity of the manufacturing during different phases of production. Furthermore, the industrial competitiveness and sustainability in EU will be strengthened.
Status
CLOSEDCall topic
FoF-08-2015Update Date
27-10-2022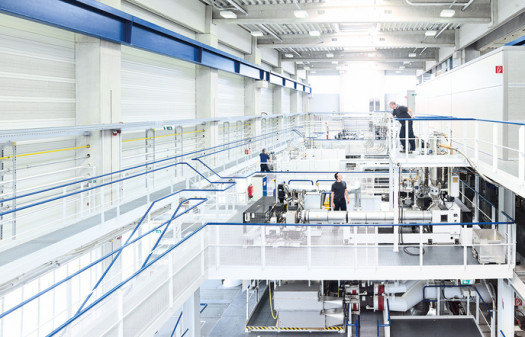
Factories of the Future Partnership - Made in Europe Partnership
The operator has a broad decision basis as well as a suggestion of a DSS (Decision Support System), which will improve the manufacturing system. Operator interaction will be done by a new developed HMI (Human Machine Interface) providing the huge amount of data in a reliable manner.
Optimization algorithms can find sub-optimal configurations of the plant and improve them. Newly calculated system parameters are verified in a simulation before they are applied to the real plant.
Optimization algorithms can calculate better plant configurations. Thesting these new configurations in a real plant can be very cost intensive as production may be compromised during configuration and testing. A virtual environment which is able to simulate new parameters and verify them is a big deal as the running production is not compromised during testing and evaluation of new parameters.
The main focus of IMPROVE are learned models. Manual modeling of system models is not suitable for the complex, fast chaning industrial plants we have today. Lots of expert knowlege is needed to manually create a model. Learned models can be created using only data and little to no expert knowledge is required depending on the technology.