The Whirlpool testbed is focused on the foaming process, the core process in fabricating refrigerators and one of the most complex fabrication processes in the White Goods industry. In particular, the Whirlpool demonstrator is focused on the prediction of the behaviour of the injection head of a polyurethane foaming machine. The current maintenance of the equipment is based on a preventive structure that is planned at regular time intervals. The main scope of the demonstrator is to identify and validate a mechanism of health prediction through the correlation of sensor data to alarms recorded at the machine level, which are good indicators of severe problems upcoming on the injection head. The main target is to develop a RUL (Remaining Useful Life) estimator and thus allow the maintenance organization to schedule substitution of the injection head just before its irreversible damage. This can positively impact the business: reducing unexpected breakdowns and impacting OEE; reducing maintenance cost, and improving main maintenance KPIs (MTTR and MTBF).
WHR expectation from the demonstrator:
- Evaluate and validate analytical strategy to extract as much possible useful information from available data
- Evaluate the robustness of the technical solution to ensure good trust in the system from the operators
- Evaluate the effectiveness of data visualization platform
- Evaluate the effectiveness of RUL estimation
- Evaluate the AR support tool to maintenance operator
The White Goods demonstrator includes a SERENA system deployment on the cloud and a virtual gateway in charge of collecting and pushing data to the SERENA cloud platform. The most critical aspect is predictive analytics which includes the offline dynamic and evolvable building and training of the predictive model along with the periodic prediction task evaluating the real-world data and generating a prediction label along with a RUL value. In greater detail, machine’s sensors are available to the SERENA platform through an SQL database connected with the already available SCADA system. A gateway provides a robust and secure way to transfer the data in RT from the factory DB to the SERENA platform using a VPN provided by Whirlpool. Data Visualization and Analytics were provided back to operators via the SERENA platform.
Whirlpool aims to reduce the burden on maintenance by ensuring maximum machine availability which will, in turn, reduce unnecessary breakdown thereby extending the life of components. SERENA contributed to an increase to OEE by 5.5%, a 15% reduction of MTTR, an increase of 100% to the MTBF and an estimated reduction of TCM by €8.000.
Country: | IT |
Address: | Via Aldo Moro, 5, Biandronno 21024 |
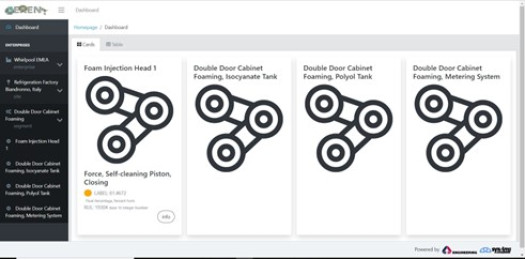
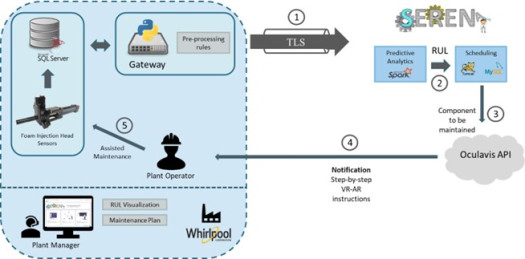
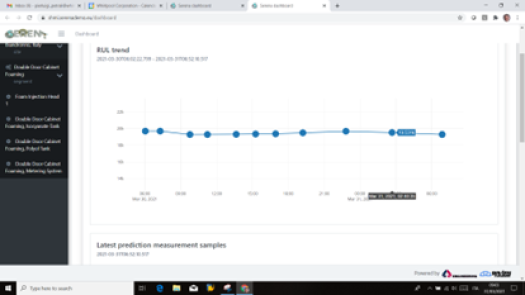
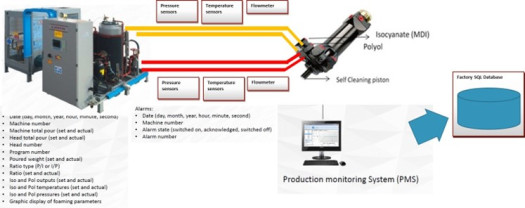
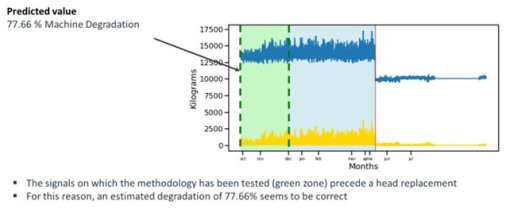
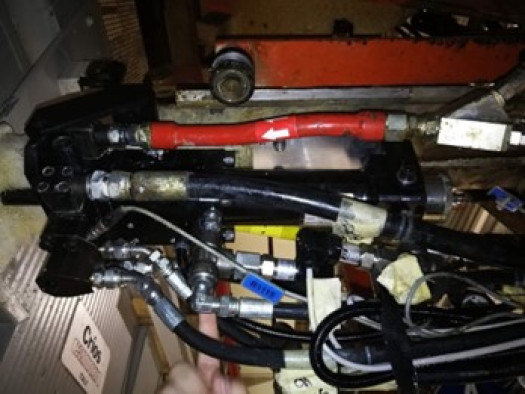
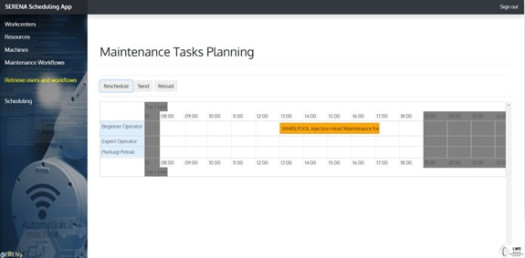
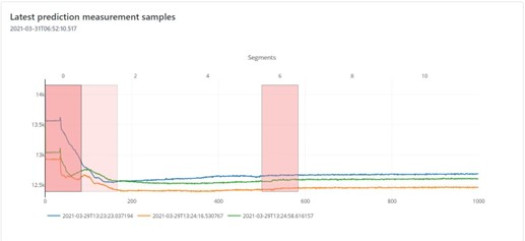
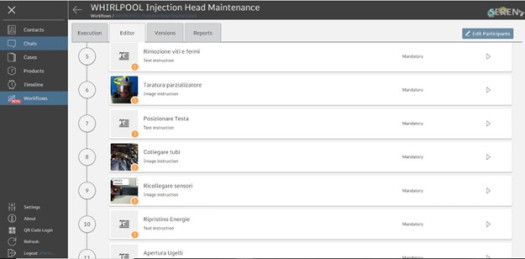
All the experiments conducted have been interpreted within their business implication: a reliable system (i.e. robust SERENA platform and robust data connection) and an effective Prediction System (i.e. data analytics algorithm able to identify mixing head health status in advance) will have an impact on main KPI related to the foaming machine performances. In particular:
Besides practical results, SERENA provided some important lesson to be transferred into its operative departments:
Data Quality
Finding the relevant piece of information hidden in large amounts of data, turned to be more difficult than initially thought. One of the main learnings is that Data Quality needs to be ensured since the beginning and this implies spending some more time, effort and money to carefully select sensor type, data format, tags, and correlating information. This turns particularly true when dealing with human-generated data: if the activity of input data from operators is felt as not useful, time-consuming, boring and out of scope, this will inevitably bring bad data.
Some examples of poor quality are represented by:
a. Missing data
b. Poor data description or no metadata availability
c. Data not or scarcely relevant for the specific need
d. Poor data reliability
The solutions are two: 1) train people on the shop floor to increase their skills on Digitalization in general and a Data-Based decision process specifically; 2) design more ergonomic human-machine interfaces, involving experts in the HMI field with the scope of reducing time to insert data and uncertainty during data input.
These two recommendations can bring in having a better design of dataset since the beginning (which ensure machine-generated data quality) and reduce the possibility of errors, omissions and scarce accuracy in human-generated data.
Data Quantity
PU foaming is a stable, controlled process and it turned to have less variation: thus, machine learning requires large sets of data to yield accurate results. Also, this aspect of data collection needs to de designed in advance, months, even years before the real need will emerge. This turns into some simple, even counterintuitive guidelines:
1. Anticipate the installation of sensors and data gathering. The best is doing it at the equipment first installation or its first revamp activity. Don’t underestimate the amount of data you need to improve good machine learning. This, of course, also needs to provide economic justification since the investment in new sensors and data storing will find payback after some years.
2. Gather more data than needed. Common practice advice is to design a data-gathering campaign starting from the current need. This could lead, though to missing the right data history when a future need emerges. In an ideal state of infinite capacity, the data gathering activities should be able to capture all the ontological descriptions of the system under design. Of course, this could not be feasible in real-life situations, but a good strategy could be to populate the machine with as many sensors as possible.
3. Start initiatives to preserve and improve the current datasets, even if not immediately needed. For example, start migrating excel files spread in individuals’ PC into commonly shared databases, making good data cleaning and normalization (for example, converting local languages descriptions in data and metadata to English).
Skills
Data Scientists and Process Experts are not yet talking the same language and it takes significant time and effort from mediators to make them communicate properly. This is also an aspect that needs to be taken into account and carefully planned: companies need definitely to close the “skills” gaps and there are different strategies applicable: train Process Experts on data science, train data scientists on the Subject matter; develop a new role of Mediators, which stays in between and shares a minimum common ground to enable the extreme cases to communicate.