The Predictive Maintenance demonstrator is focused on the monitoring of the operation of an edge banding machine to detect anomalous working conditions evaluating the risk of machine breakdown and take preventive actions through machine data analysis.
This experimentation has been deployed in a real manufacturing environment in the Spanish company LAGRAMA. They produce high-quality home furniture and they are located in Vinaròs (Spain).
The operator gets live information from the UI, which is accessible from the portal. The operator can monitor information, such as the failure prediction and confidence in the prediction. Sensors readings can be monitored from the UI as well to help identify the location of a fault. Working in batches means many machine stops and, of course, affects whether a machine is running all the time continuously. Predicting when the edge banding machine may need maintenance of a part or motor is a significant advance for LAGRAMA since it can prevent any part from being damaged, decreasing productivity and consequently losses and a bad image in the face of customers for late deliveries
To predict when the edge banding machine requires some maintenance operation, machine data is captured from the machine by retrofitting sensors (temperature, electric current and air pressure) to parts of the machine.
The sensors are connected to an interface board on the Industreweb Factory Connector, which monitors the values and publishes them to the EFPF Data Broker. The Analytics component monitors these data and builds up a machine learning model. This enables the publication of a special event to the Message Broker when the machine operation values indicate some potential problem.
This way, the Risk component, which is subscribed to the Analytics Component's output topic, can evaluate if there is a risk of a machine breakdown and, in such case, this component triggers an alert to maintenance staff in LAGRAMA.
In summary, we can conclude that the value proposition for LAGRAMA is:
- Reduction of the reaction time when some problem arises during production
- Reduction of the machine downtime due to primary failure in the machine
- Avoiding failures that may affect the quality of produced goods through the detection of abnormal machine operation.
Web resources: | https://www.efpf.org/ |
Country: | ES |
Address: | Polígono Vinaròs Ulldecona Km 11, Carrer Planes Altes A, Vinaròs 12500 |
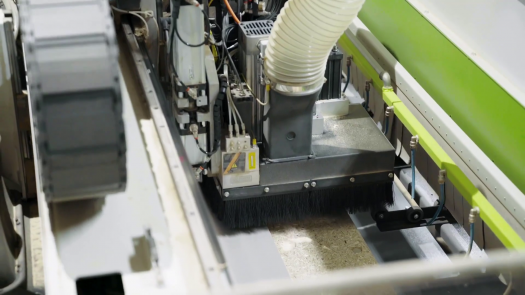
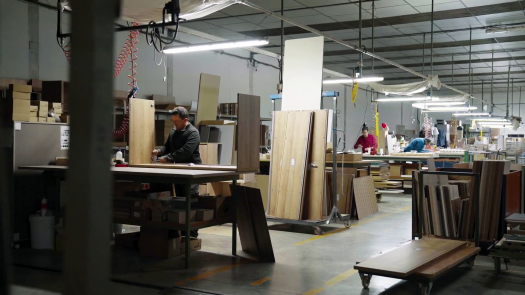
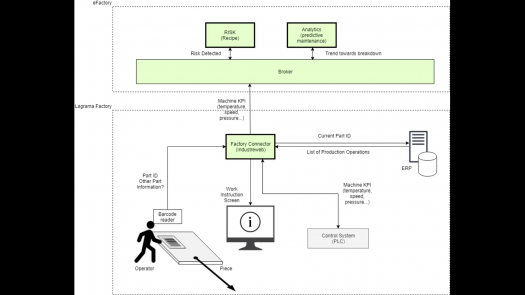
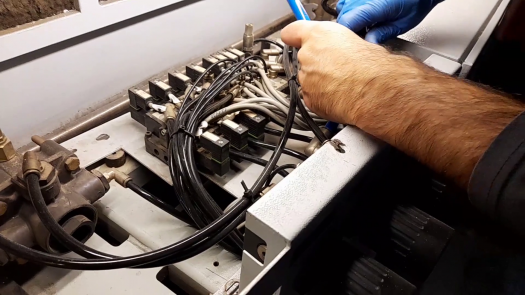
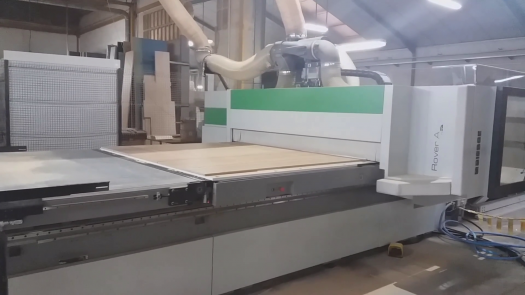
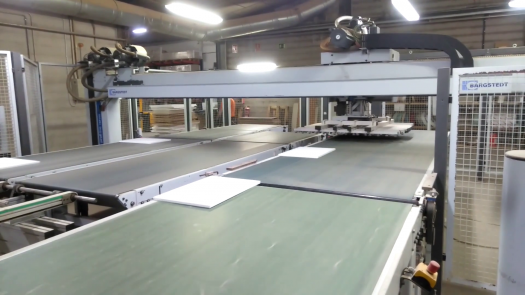
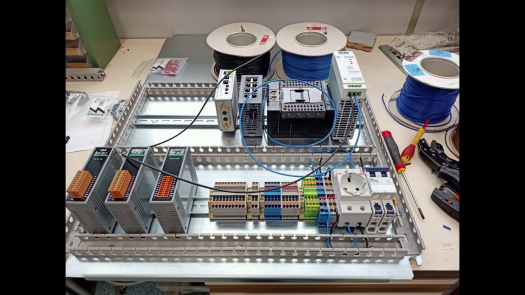
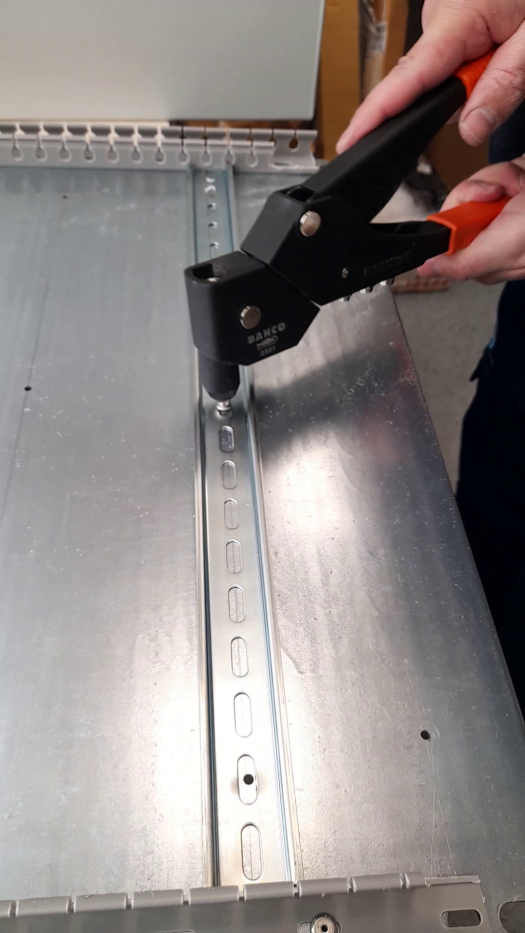
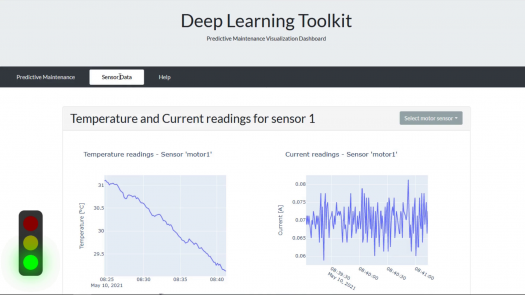
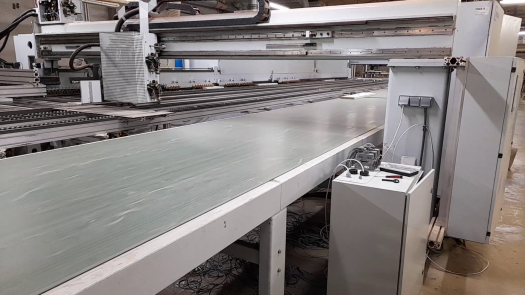
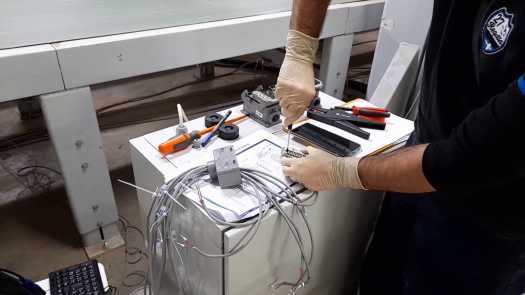
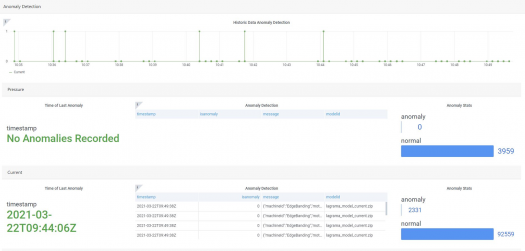
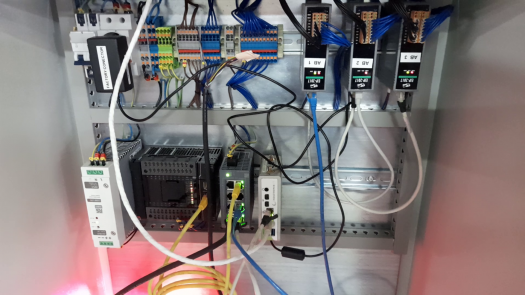
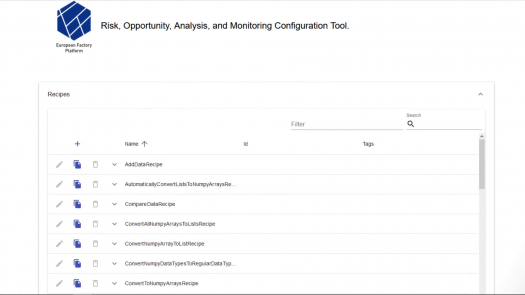