Summary
The experiment, conducted by COMET, is about IDSS FOR PREDICTIVE QUALITY ASSURANCE.
COMET decided to focus its experiment on quality control. Advanced technology enabled quality control can be applied in various industries by leveraging e.g. big data analytics and smart sensors and, of course, AI. Such predictive quality system performs cause-effect analysis, big data monitoring and analytics, and effective decision-making in real time.
The underpinning idea is to provide local manufacturing companies with an use case, a pilot experiment, easily replicable and scalable, that might demonstrate the early identification of defects and failures in larger series production. Early estimating the potential faultiness could help local manufacturing companies on planning, controlling and executing productive activities in an optimized and predictive manner. The scope of investigation is of particular relevance in large-series production e.g. for local Tier 2 companies in the automotive sector, steel producing companies and metal industry suppliers, manufacturing enterprises producing anchors and wall plugs, etc... In this context, the idea was to focus on an intelligent system that, leveraging on machine learning, could be of help to the decision maker (i.e. the quality assurance manager) to identify and detect the defects, anomalies and outliers (i.e. faulty pieces) in an environment similar to the real one.
One local manufacturing SME producing components in big lots was involved in the experiment, providing the faulty pieces that will be the object of the investigation. Large amounts of data have been the raw material on which the system relied on, as AI technology make it possible to identify regular patterns in available data and to use these patterns for a wide variety of analysis, decision-making, and prediction purposes.
COMET belief is that an intelligent learning approach such as Machine Learning should be investigated and compared for manufacturing quality prediction. The use case will leverage on an intelligent approach, part of a broader family of learning methods based on artificial neural networks with representation learning. In the age of industry 4.0, machine and deep learning has attracted increasing interest for various research applications. In recent years, such AI models have been extensively implemented in fault detection and diagnosis systems. The machine architecture’s automated feature learning process offers great potential to solve problems with traditional fault detection and diagnosis systems.
The company involved in the experiment was an Italian enterprise, dealing with cold moulding of metal wires for the production of small steel components to be employed in in the automotive and furniture sectors.
The use case implementation is based on different steps:
-
first of all, a number of sensors were installed on the first part of the printer-line, in order to detect, since the beginning, possible defects and stopping the production of a low-quality component;
-
then, a MES was installed and it was the basis of the AI-based decision support system developed by COMET, but the installation was not part of the Experiment and it was performed by a third party;
-
finally, data collected were exploited to implement an AI-based system for fault detection.
More information & hyperlinks
Country: | IT |
Address: | Via Roveredo 20B, Pordenone 33170 |
Images
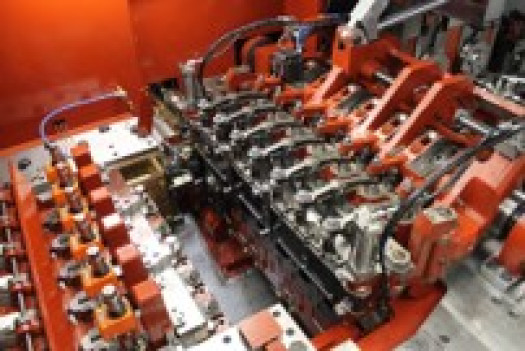
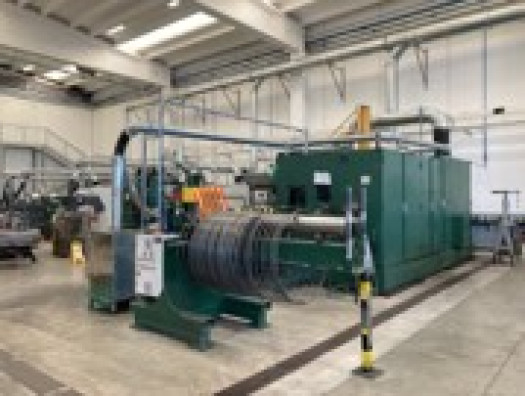
Geographical location(s)
Structured mapping
Unfold all
/
Fold all
Comment:
Early estimating the potential faultiness could help local manufacturing companies on planning, controlling and executing productive activities in an optimized and predictive manner.
Production planning optimization
Reduced costs
Optimized efficiency and effectiveness of the manufacturing systems
(European) Digital Innovation Hubs (EDIH)
European Digital Innovation Hubs Cases and Demonstrators (DIH)
Automation and integration of the manual Quality Control (QC)
Objectiveness and precision of the QC
Productive increase of QC supervisors