MAShES proposed a breakthrough approach to image-based laser processing closed-loop control. Firstly, a compact, snapshot, and multispectral imaging system in the VIS/MWIR spectral range was developed. This approach enabled a multimodal process observation that combines different imaging modalities. Moreover, it enabled an accurate estimation of temperature spatially resolved and independent on emissivity values, even for non-grey bodies. Secondly, a fully embedded approach to real time (RT) control was adopted for efficient processing of acquired data and high speed -multiple inputs/ multiple outputs- closed-loop control. Thirdly, a cognitive control system based on the use of machine learning techniques applied to process quality diagnosis and self-adjustment of the RT control was developed.
As a result, a unified and compact embedded solution for RT-control and high speed monitoring was developed that brings into play:
- The accurate measurement of temperature distribution,
- The 3D seam profile and 2D melt pool geometry,
- The surface texture dynamics, and process speed.
MAShES control act simultaneously on multiple process variables, including laser power and modulation, process speed, and spot size. MAShES also delt with usability and interoperability issues for compliance with cyber-physical operation of the system in a networked and cognitive factory. Moreover, standardisation issues were addressed regarding the processes and the control system . MAShES system was designed under a modular approach, easily customizable for different laser processing applications in highly dynamic manufacturing scenarios. Validation and demonstration of prototypes of MAShES system were done for laser welding and laser metal deposition (LMD) in operational scenarios at representative end-user facilities.
Web resources: |
http://www.mashesproject.eu
https://cordis.europa.eu/project/id/637081 https://www.youtube.com/channel/UCQp4Ubw_XB4OiTWT9vQLhzQ - You Tube channel |
Start date: | 01-12-2014 |
End date: | 30-11-2017 |
Total budget - Public funding: | 3 673 157,00 Euro - 3 673 157,00 Euro |
Original description
MAShES proposes a breakthrough approach to image-based laser processing closed-loop control.Firstly, a compact, snapshot, and multispectral imaging system in the VIS/MWIR spectral range will be developed. This approach will enable a multimodal process observation that combines different imaging modalities. Moreover, it will enable an accurate estimation of temperature spatially resolved and independent on emissivity values, even for non-grey bodies and dissimilar materials. Secondly, a fully embedded approach to real time (RT) control will be adopted for efficient processing of acquired data and high speed -multiple inputs/ multiple outputs- closed-loop control. Thirdly, a cognitive control system based on the use of machine learning techniques applied to process quality diagnosis and self-adjustment of the RT control will be developed.
As a result, a unified and compact embedded solution for RT-control and high speed monitoring will be developed that brings into play:
- The accurate measurement of temperature distribution,
- The 3D seam profile and 2D melt pool geometry,
- The surface texture dynamics, and process speed.
MAShES control will act simultaneously on multiple process variables, including laser power and modulation, process speed, powder and gas flow, and spot size.
MAShES will deal with usability and interoperability issues for compliance with cyber-physical operation of the system in a networked and cognitive factory. Moreover, standardisation issues will be addressed regarding the processes and the control system and contributions in this regard are envisaged.
MAShES will be designed under a modular approach, easily customizable for different laser processing applications in highly dynamic manufacturing scenarios. Validation and demonstration of prototypes of MAShES system will be done for laser welding and laser metal deposition (LMD) in operational scenarios at representative end-user facilities.
Status
CLOSEDCall topic
FoF-01-2014Update Date
27-10-2022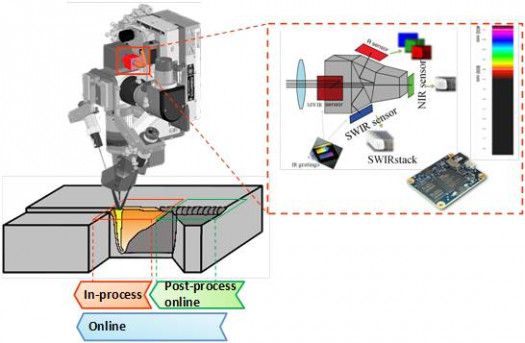